올해 초에 읽었던 논문인데, 다른 곳에 정리해 두었던 것을 발견하여 옮겨왔습니다.
당시에 파일 검색 관련 주제에 관심이 있어서 읽어보았던 Dataset search 에 대한 survey 논문입니다.
읽은 날짜 2023.02.21
카테고리 #DatasetSearch, #InformationRetrieval
Dataset search: a survey
- Authors: Adriane Chapman, Elena Simperl, Laura Koesten, George Konstantinidis, Luis-Daniel Ibáñez-Gonzalez, Emilia Kacprzak, Paul Groth
- DOI: https://doi.org/10.1007/s00778-019-00564-x
- Keywords: Dataset search, Dataset retrieval, Dataset, Information search and retrieval
- Published Date: August 2019
- Publisher: The VLDB Journal (The International Journal on Very Large Data Bases)
ABSTRACT
배경 > 문제점 > 목표(RQ) > 방법 > 평가 > 결과 > 의의
[배경] 데이터셋에서 가치를 찾기 위한 검색 관련 분야가 발전하고 있으므로, 연구 분야에서 ‘dataset search’ 또는 ‘data retrieval’ 이 떠오를 것으로 예상됨.
[목표] 최신의 연구내용 및 상용 시스템을 살펴보고 ‘dataset search’ 자체를 하나의 연구분야로 만드는 것에 대한 논의 진행
[방법] information retrieval, databases, entity-centric, tabular search 에서의 데이터 셋 검색 접근 방법및 구현에 대해 조사
[의의] 질문에 대한 가능한 경로를 식별하고, 다음 단계 제시
1_ INTRODUCTION
● Dataset search 에 대한 중요성
- 의사 결정에서 Data를 사용하는 경우가 매우 늘었음.
- dataset을 공유하고 활용하는 경우도 많아졌음.
- data markets에서의 trade, open data portals에서의 dataset 공유
- Wikidata, Linked Open Data Cloud 등에서 데이터를 수집
- Intelligent assistants, recommender systems, search engine optimization 등에서의 dataset 활용
- 원칙에 따른 데이터 검색에 대한 연구는 오래 되었지만, Dataset 검색에는 아직 미결 문제가 많음.
- 현재 사용 가능한 데이터셋, 사용자에게 필요한 데이터셋, 사용자가 실제로 찾고 신뢰할 수 있고 사용할 수 있는 데이터셋 에는 단절이 있다.
- available | user needs | user can actually find& trust
● 기존 Dataset search 의 한계
- dataset search는 주로 published metadata에 대한 keyword-based 기반임.
- 문제점:
- (1) 사용 가능한 메타데이터는 사용자가 데이터 세트가 주어진 작업에 적합한지 평가하는 데 필요한 실제 정보를 포함하지 않을 수 있다.
- -Available metadata may not encompass the actual information a user needs to assess whether the dataset is fit for a given task [106].
- Koesten, L.M., Kacprzak, E., Tennison, J.F.A., Simperl, E.: The trials and tribulations of working with structured data: a study on information seeking behaviour. In: Proceedings of the 2017 CHI Conference on Human Factors in Computing Systems, Denver 2017, pp. 1277–1289 (2017). https://doi.org/10.1145/3025453.3025838
- (2) 검색 결과는 웹 기반 정보에 대해 작동하는 필터 및 경험을 기반으로 사용자에게 반환되지만 항상 데이터 세트로 잘 전송되지는 않는다.
- Search results are returned to the user based on filters and experiences that worked for web-based information, but do not always transfer well to datasets [68].
- 이런 문제점은 검색된 데이터의 사용에 영향을 미침.
- 머신러닝은 dataset에 대해 수행된 처리에 영향을 많이 받을 수 있으나,
- 데이터를 수집하는 원래 목적은 데이터를 해석하고 분석하기 위함인데, 기존의 데이터셋 검색 방식은 이를 제대로 반영하지 못했음.
- These limitations impact the use of the retrieved data—machine learning can be unduly affected by the processing that was performed over a dataset prior to its release [168], while knowing the original purpose for collecting the data aids interpretation and analysis [185].
● Dataset search 의 개선 방향
- dataset search 컨텍스트에서의 접근법에는 다음과 같은 aspects가 고려되어야 한다.
- data provenance (출처)
- data annotations (주석) //description을 뜻하는 듯 함.
- data quality
- granularity of content
- schema
- 사용자는 대용량 데이터를 살펴볼 여력이 되지 않기 때문에, 그들의 관심사가 제1순위로 고려되어야 한다.
- 경우에 따라, 다양한 소스에서의 데이터를 통합하여 새로운 데이터셋으로 구축할 수 있어야 한다.
- 앞으로 우리가 generating, maintaining, releasing 할 데이터셋의 잠재력을 실현하기 위해 더 많은 연구가 필요함.
● 페이퍼 구성 및 주요 내용
- 데이터셋 검색은 다른 여러 관련 분야에 기반을 두고 있으며, 아래 섹션들에서 다룸.
- Section 2 - 기본적인 dataset search problem review
- Section 3 - commercial dataset search offerings
- Section 4 - overview of ongoing dataset search research
- Section 5 - open problems
- Section 6 - discussion
2_ BACKGROUND
● Dataset
- dataset의 정의는 매우 추상적이며, 커뮤니티에 따라 각자 다른 정의를 허용함.
- 이 페이퍼에서는 dataset이란 특정 목적을 위해 형식화 되고 조직화된 observations 의 콜렉션으로 정의함.
- (A collection of related observations organized and formatted for a particular purpose. )
- dataset은 테이블 형태의 자료 이외에 이미지, 그래프, 문서일 수 있다.
● Dataset search
- dataset search 는 discovery, exploration, 그리고 결과 dataset 리턴 을 포함한다.
- 이 문서에서는 alphanumeric data(텍스트, 엔티티 데이터) 로 한정함.
● Dataset search Types
(1) basic dataset search (기본적인 데이터셋 검색)
- 관찰된 관련 자료들이 특정 목적에 맞게 조직화 되어 소비/재사용 되기 위해 공개된 것. (the set of related observations are organized for a particular purpose and then released for consumption and reuse. )
- Individual data repositories (개별 데이터 저장소) 내에서 이런 인터랙션 패턴을 볼 수 있다.
- Research data: Figshare, Dataverse, Elsevier Data Search
- Open data portals
- Search engines: DataMed, Google Dataset Search
- Example 1 (Basic dataset search)
- Hurricane Sandy가 뉴욕시의 gasoline prices에 미친 영향에 대한 기사를 쓰는 상황.
- 참고할 수 있는 데이터셋 A, B 모두 Hurricane Sandy 이후의 뉴욕시 gasoline 가격을 나타냄.
- dataset A: the American Automobile Association (AAA)
- dataset B is from Twitter.
- 어느 데이터셋을 사용할 것인가는, 사용자의 tool-set이나 데이터 리터러시(이해도) 뿐만 아니라, 필요한 정보 요구의 세부사항, 알고리즘 또는 처리 방법에 따른 요구사항이나 목적에 달려있다.
- (The choice of which dataset to use depends on the specifics of the information need, potentially the purpose and requirements of algorithms or processing methods, as well as the user’s tool-set and data literacy. )
- 적합한 데이터셋을 찾기 위하여, 사용자는 데이터셋을 반환하는 ‘query’ 를 실행해야 하고, 각자 요구사항에 맞게 데이터셋에서 내재된 차이점에 따라 우선 순위를 변경해야 한다.
- (In order to find the right dataset, a user must issue a query that will return datasets, not tuples, documents or corpora. Differences inherent in the datasets should alter their ranking. )
- 동일한 content를 기반으로 결과를 필터링 하지만, 사용자마다 다른 기준과 지표를 사용하여 데이터셋의 순위를 매긴다.
- 쉬운 데이터를 원한다면 → A dataset
- 정확한 타임라인이 필요하다면 → B dataset
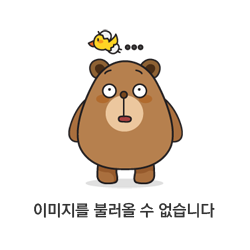
(2) Constructive dataset search (구조적/구성적 데이터셋 검색)
- Dataset search 에는 검색하는 사람이 특정 목적을 위해 구성한 관련 observations의 세트를 포함할 수 있다.
- 관련 행동 패턴이 보이는 사례
- data lakes
- data markets
- tabular search
- Example 2 (Constructive dataset search)
- 브라질 리우데자네이루의 한 도시는 홍수를 예방하고 관리하기 위한 전략을 개발하는데 data-informed approach를 따르고자 함.
- 수집 데이터
- raffic & public transport; utility and emergency services; weather; citizen reports;
- 정보 활용 시나리오
- weather 데이터셋에서 강수량이 돌발 홍수를 유발함 ⇒ traffic volume 데이터와 통합 ⇒ 비상 대응상황 식별하는 것으로 보강됨 ⇒ 이벤트 발생동안 위험에 처한 인구 강조
- RapidMiner 라는 extension에서 이와 같은 데이터셋의 생성 및 예시 제공
- (참고 자료)
RapidMiner | Amplify the Impact of Your People, Expertise & Data
The RapidMiner platform amplifies the impact of people, expertise & data for breakthrough competitive advantage, no matter where you are in your data science journey.
rapidminer.com
2.1_ Overview of dataset search
- 검색 프로세스에 대한 개략적인 구조 및 관련 커뮤니티에서 연구된 주제에 대한 주요 프로세스 단계 매핑
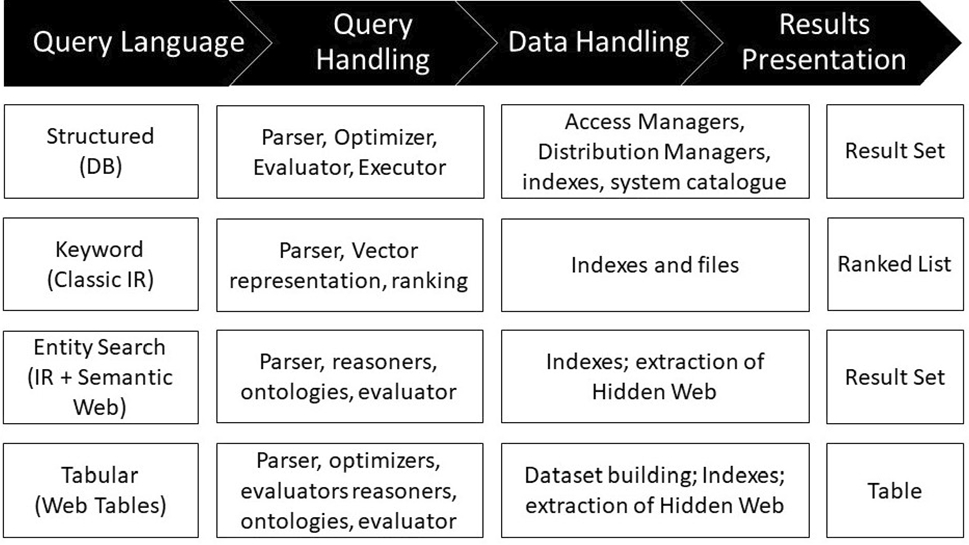
- dataset search에 대한 일반적인 접근 방식은 기존의 키워드 기반 정보 검색 시스템에 대한 사용자의 경험을 모델링 하는 것이다. 즉, 사용자가 쿼리를 제시하면, 순위가 매겨진 데이터셋의 리스트가 반환 되는 방식이다.
- 아래 그림(Figure 3): UK government’s open data portal의 검색 인터페이스
- keyword 검색 박스 - query 제시
- “Filter by” 박스 - 이미 정의된 카테고리 내에서 data의 subset(하위집합)을 지정할 수 있다.
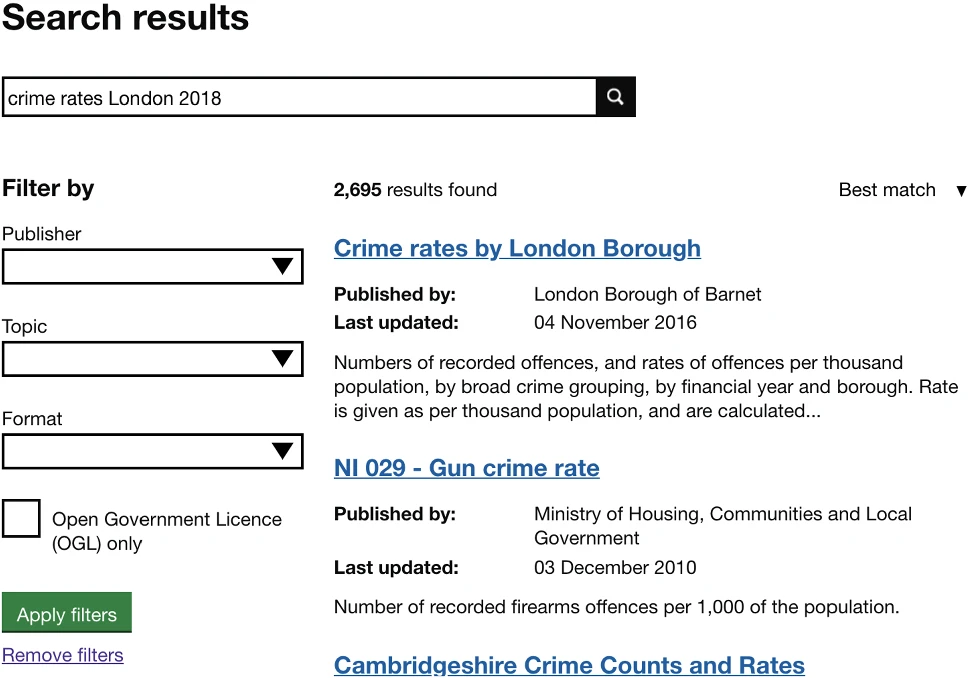
● Querying
- dataset search에서 ‘query’: 일반적으로 keyword 또는 Contextual Query Language (CQL) 표현
- 이 페이퍼에서는 ‘query’ 라는 용어를 사용하여, 각 분야의 검색 기술에 대해 서술할 때 특정 기술에 대한 semantically(의미적), syntactically(구문적, according to syntax) 올바른 검색 표현을 의미한다.
- 데이터베이스에서 query는 SQL (Structured Query Language)
- 정보 검색(information retrieval)에서 query는 CQL (Contextual Query Language)
● Query handling
- 사용자가 제출한 정보는 dataset에 대한 메타데이터를 검색하는 데 사용된다.
- search term과 메타데이터가 유사한 정도에 따라 결과가 생성된다.
● Data handling
- Publishers는 데이터셋에 대한 메타데이터(title, description, language, temporal coverage, etc.) 를 채워넣는다.
- 메타데이터에 쓰이는 어휘(vocabularies)
- DCAT, schema.org 또는 CSV on theWeb 과 같은 어휘를 출발점으로 사용할 수 있다.
- 목적: 모든 파일에 대해 데이터의 타입이나 포맷을 일관되게 제공하여 validation 의 기반을 제공하고, 잠재적인 에러를 방지하기 위함. (The goal of these vocabularies is to provide a uniform way of ensuring consistency of data types and formats (e.g., uniqueness of values within a single column) for every file, which can provide basis for validation and prevent potential errors. )
- sensemaking을 위해 데이터셋에 descriptions를 추가하기도 함.
- 한계:
- data handling은 수동으로 진행되므로 resource-intensive(리소스 집약적)이며 따라서 데이터셋의 description이 충분하지 않거나, 충분한 세부 정보가 포함되지 않는 경우가 많다.
- Search term과 query를 매칭하는 Query handling의 방법과 기능을 제한
● Results presentation
- SERPs (Search Engine’s Results Pages, 검색 엔진의 결과 페이지) 는 전통적으로 10 blue links 패러다임을 따른다.
- (참고 자료)
10 blue links: are they dead or alive in search?
It’s a provocative question and heaven knows we all love one of those. There are a lot of contradictory opinions out there surrounding the term ‘blue links’ and how many are to be found on your…
econsultancy.com
- Dataset search에서 기본 filtering 옵션은 faceted search 에 사용할 수 있다.
- 검색 결과를 클릭 → 일반적으로 메타데이터, a free-text summary 또는 데이터 미리보기 화면으로 이동함.
- Google Dataset Search의 경우, 검색 결과를 분할 인터페이스로 표시
- 왼쪽: 스크롤 가능한 검색 결과 목록
- 오른쪽: 하나 (또는 여러개)의 리포지토리의 dataset preview page의 축소된 버전
2.2_ Common search architectures
● 데이터셋 검색 동작 방식
- 데이터셋 검색은 local 또는 global 하며, Distributed database 와 유사한 방식으로 동작한다.
- Dataset에 대한 Query가 주어지면, Query engine은 먼저 query에 관련된 적절한 데이터셋을 고른 후, 다양한 접근 방법 중에서 선택한다.
- aggregating the datasets locally (데이터셋을 로컬에서 집계하거나)
- using distributed processing as in Hadoop (하둡과 같은 분산 시스템을 사용하거나)
- query federation (쿼리 연합)
- (참고 자료): query federation을 사용하면, 클라우드 데이터베이스 (예: data warehouse, data lake 등.) 에서 다양한 데이터 소스에 연결하여 클라우드 DB 데이터와 Join 하여 임시 테이블로 가져올 수 있다.
- (In a similar manner to a distributed database, given a query Q and a set of datasets (the sources), the query engine first selects the datasets relevant to the query [160,177] and then chooses between different approaches: aggregating the datasets locally, using distributed processing as in Hadoop [188], or query federation [143]. )
● 데이터셋 검색 문제
- 데이터셋 검색 문제는 다양한 수준에서 해결될 수 있다.
- (1) Global level 문제
- Google Dataset Search, DataMed와 같은 서비스
- 웹을 크롤링 하고 모든 분산 리소스에서 글로벌 검색을 용이하게 함.
- 이런 방식은, 메타데이터 마크업에서 발견한 tags를 사용하여 메타데이터가 데이터셋에 중요한지를 식별하고 구조화한다.
- 이 tags들은 from schema.org 또는 DCAT의 단어를 활용하여 표현된 것 이어야 한다.
- Google Dataset Search, DataMed와 같은 서비스
- (2) Local level 문제
- open government data portals (예: data.gov.uk) , 조직의 data lakes, Elsevier’s 와 같은 과학 리포지토리, 또는 data markets
- 사용자는 특정 목적을 위한 데이터셋을 찾고 평가하고자 한다.
- 이런 사용자를 지원하려면 데이터를 인풋 형식으로 지정하고, 데이터 전문가의 요구사항을 특정 정보로 고려할 수 있게 하는 프레임워크, 방법, 툴이 요구된다. (Supporting them requires frameworks, methods and tools that specifically target data as its input form and consider the specific information needs of data professionals.)
- open government data portals (예: data.gov.uk) , 조직의 data lakes, Elsevier’s 와 같은 과학 리포지토리, 또는 data markets
2.3_ Other search sub-communities
- 데이터 타입, 사용된 방법에 따라 다양한 검색 시나리오가 해결되어왔다.
- 관련하여 하위 분야(subdisciplines)로는 databases, document search (class information retrieval), entity-centric search (시맨틱 웹의 컨텍스트에서 다루어짐, database와 information retrieval에서의 knowledge discovery), 그리고 tabular search (광범위한 데이터 매니지먼트, IR 및 종종 entity-centric search).
- Figure 2에는 이런 하위 분야에서 핵심 검색 기능을 구현하기위해 사용했던 방법들이 나와있다.
- Query Language (writing) > Query Handling > Data Handling > Results Presentation
- Dataset search는 그 자체로도 독특한 챌린지와 성격을 갖는 하나의 연구 분야 이지만, 이런 모든 분야에서 commonalities(공통점)을 공유하고 인사이트를 얻는다.
- 이 섹션에서는 각 커뮤니티 별 포커스와 툴을 리뷰한다.
- 특히 underlying data에서와 동일한 타입의 오브젝트가 리턴이 되는 경우에 주목한다. 예를 들어, 데이터베이스 데이터에서 결과세트에서 데이터, 도큐먼트 코퍼스에서의 도큐먼트 하나…)
- 추가적인 프로세싱과 reasoning steps를 포함하는 question answering과 같은 접근법은 무시한다.
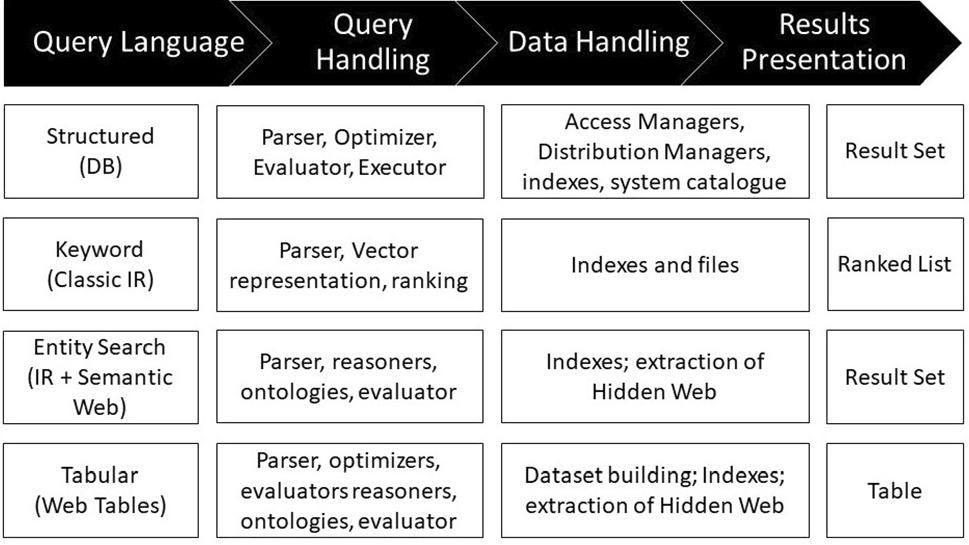
2.3.1_ Databases
● 데이터베이스 검색 파이프라인
- 고전적인 방법
- Structured query (구조화된 쿼리) > Parsing the query (쿼리 파싱)> Creating an evaluation plan (평가 계획 수립) > Optimizing the plan (계획 최적화) > Executing the plan utilizing appropriate indexes and catalogues. (적절한 인덱스와 카달로그를 활용하여 계획 실행)
- 최근에 추가된 작업:
- 웹의 숨겨진 영역에서 더 많은 데이터를 발견 (uncover more data from hidden areas of the web: hidden/Deep web search)
- HTML로 작성된 웹 형식 “뒤에” 있는 콘텐츠
- 의료 연구 데이터, 금융 정보, 쇼핑 카탈로그 등
- 웹 크롤러가 직접 엑세스 할 수 있는 surface web 보다 콘텐츠 규모가 훨씬 큰 것으로 추정
● 딥 웹에서 데이터 검색 방법
- 접근 방식 1 (전통적인 기술 - vertical search engine)
- vertical search engine 구축 → 각 웹사이트와 특정 도메인에 최적화 된 중앙 중재자(centralized mediator) 사이에 semantic mappings 구성
- structured queries는 mediator에 제시됨 → mappings통해 웹에 리다이렉트 됨.
- 예: Kosmix
- vertical engines for a large number of domains (health, scientific data, car sales etc.)
- (참고 자료)
- Kosmix: high-performance topic exploration using the deep web
- https://dl.acm.org/doi/10.14778/1687553.1687581
- 접근 방식 2 (결과 웹페이지를 생성)
- form의 possible input을 학습하고, 중앙의 매개 형식을 생성한다.
- Google - 다양한 언어로 작성되고 수백 개의 도메인에 걸쳐있는 수백만 HTML형식의 입력을 자동으로 추정하고, 결과 HTML페이지를 search engine index에 추가하였음.
- A second group of approaches tries to generate the resulting web pages, usually in HTML, that come out of web form searches. Google has proposed a method for such surfacing of deep web content by automatically estimating input to several millions of HTML forms, written in many languages and spanning over hundreds of domains, and adding the resulting HTML pages into its search engine index [128]. The form inputs are stored as part of the indexed URL. When a user clicks on a search result, they are directed to the result of the (freshly submitted) form.
2.3.2_ Information Retrieval
- IR 종류(class): 문서 검색, 웹 검색, 오브젝트 타입(이미지, 사람 등)에 따른 검색 엔진 등
- 텍스트 기반 IR - 도큐먼트의 적절한 검색어(search terms)를 계산하기 위하여, 다양한 통계적, 의미론적 기술을 사용
- 특수 검색 엔진 - 기본 리소스 특성에 맞게 조정됨.
- 특징: 특수성, 제한된 리소스 범위
- vertical search engine이 precision이 높을때가 있음.
- 더 복잡한 스키마를 활용하여 사용자의 복잡한 태스크 시나리오를 지원하고자 하는 경향
- 예: email search - 관련있는 기능을 정의하기 위하여 sender, receiver, topic, timestamp 등의 aspects고려
- 특징: 특수성, 제한된 리소스 범위
2.3.3_ Entity-centric search
- Entity-centric search information: 관심 엔티티와 해당 속성 및 관계를 통해 구성되고 액세스 됨.
- 주요 커뮤니티: Knowledge discovery, Semantic web
● Knowledge discovery
- data mining과 머신러닝을 향상시키기 위한 (centralized) 그래프 구축에 초점
- Knowledge graph(KGs) 구축
- 서로 연결된 엔티티 간의 대규모 콜렉션
- 인코딩 시, linked data를 사용하거나/하지 않거나의 경우있음.
- KGs 구축 노력: vocabularies (도메인 표현), 알고리즘(다른 소스에서의 데이터를 추출하여 그래프로 매핑), 큐레이션 및 진화
● Semantic web
- 오픈되고 분산된(decentralized)환경에서 정보의 관리에 초점
- 기계가 이해할 수 있는 그래프 기반의 데이터 표현에 집중
- 관련 연구
- 엔티티에 대한 구조화된 데이터를 제시
- 엔티티 간의 노드 연결로 분산된(decentralized) 공간에서 search와 exploration을 지원
- 온라인 검색 및 브라우징 동작과 비슷하게 하고자 함.
- RDF (Resource Description Format)
- W3C (The World Wide Web Consortium)에서 정의한 리소스 데이터의 표현 및 교환 표준 모델
- 전통적인 웹 콘텐츠(information resources), 오프라인의 엔티티(noninformation resources) 함께 포함.
- 엔티티 예: 사람, 장소, 단체 등
- IRIs (International Resource Identifiers) 로 식별됨.
- Properties: 엔티티 또는 엔티티의 attributes를 연결
- IRIs를 재사용하고 연결하여, 퍼블리셔는 동일 엔티티에 대한 데이터를 보유하고 있음을 보임. → multiple datasets에 대한 쿼리를 추가적인 integration노력 없이 가능하게 함.
- 그러기 위해서는 data가 linked data로 인코딩 되고 퍼블리쉬 되어야 함. → IRIs, RDF, RDFS(RDF Schema), HTTP 와 같은 기술과 아키텍쳐를 포함한 데이터
- To take advantage of these features, data needs to be encoded and published as linked data [9], which refers to a set of technologies and architectures including IRIs, RDF, RDFS (RDF Schema) and HTTP.
- 데이터 구조: vocabularies(단어)로 정의
- vocabularies : dataset간에 재활용 가능 - to facilitate data interpretation and interlinking
- Linked Open Vocabulary
- https://lov.linkeddata.es/dataset/lov/
- 수 백만개의 어휘에 대한 search, exploration 지원 - publishers 의 단어 검색을 도움.
- Interlinking 관련 문제점: (1)entity resolution (2)link discovery
- (1) entity resolution:
- 두 개 이상의 데이터셋에서, 어떤 엔티티와 프로퍼티가 동일한 지 식별.
- entity resolution의 일반적인 프레임워크가 정의되어있음.
- similarity metrics - 엔티티 디스크립션 비교 용도
- development of blocking techniques - 프로세스를 효율적으로 만들기 위하여, 대략적으로 유사한 엔티티를 함께 그룹화 함
- iterative approaches - 발견된 matches(쌍)을 추가 엔티티와의 similarity를 계산하기 위한 인풋 값으로 사용.
- More recent efforts have proposed iterative approaches, where discovered matches are used as input for computing similarities between further entities.
- (2) link discovery:
- 두 개의 데이터셋이 있을 때, 하나는 엔티티 간의 프로퍼티를 찾아야 함.
- property는 ‘entity resolution’ 처럼 equivalence(등가) 또는 equality(동등)하거나 ‘part-of’ 와 같이 domain-specific 하다.
- (1) entity resolution:
- linking 장점: entity-centric search를 용이하게 한다.
- Linked data facilitates entity-centric search.
- Structured language (예: SPARQL) 로 query를 표현할 수 있음.
- Structured language는 entities, entity classes, properties and values를 포함.
- Queries 는 RDF graph로 번역되고, 퍼블리쉬 될 데이터에 매칭됨. RDF로도 또한 가능. Queries are translated into an RDF graph that is matched against the published data, which is also available as RDF [197,199].
- Query들은 로컬 또는 글로벌에서 응답될 수 있음.
2.3.4_ Tabular search
- Tabular search ≠ Table search
- Table search
- sub-task of dataset search
- keyword query를 검색하면 결과가 테이블 형태로 반환됨. (예: CSV format)
- Tabular search
- 하나 이상의 테이블을 조작하고, 확장하는 것.
- 정보 요구사항들이 테이블로 표현됨.
- One of the challenges in tabular search is to answer the latent information need of the user.
- Tabular search 목적
- attribute name과 같은 특정 정보 검색
- new attributes로 테이블 확장
- 주요 태스크
- (1) Augmentation by attribute name:
- 테이블에 새로운 column name이 주어지면, 값을 채운다.
- 또는 join 할 테이블을 찾는 것. (=table extension)
- Attribute discovery
- 테이블에 추가할 수 있는 column 찾기
- Augmentation by example
- 테이블에서 누락된 값(missing value) 채워넣기. (*imputation, 결측값 대체)
- 통합(union)될 수 있는 테이블 찾기와 유사 (= table completion)
- (1) Augmentation by attribute name:
● Table extension
- Constrained table extension
- 미리 정의된 attribute name 에 의한 테이블 확장
- Unconstrained table extension
- 테이블에 미리 정의되지 않은 label을 attribute으로 하는 새로운 칼럼추가
- constrained table extension + attribute discovery 작업으로 생각할 수 있음.
- 일반적인 방법: table similarity 을 통해 기존 테이블 탐색
- EXTEND operator: input table과 유사한 web table 발견
- Similarity: 테이블의 스키마와 관련하여 계산됨.
- 가장 유사한 테이블의 값을 사용해 입력 테이블의 추가적인 column을 채움.
- The Infogather system:
- input table과 potential augmenting tables를 단순히 계산하는 대신, 추가할 잠재적인 테이블의 주변 이웃도 고려한다.
- 이런 indirect tables는 보조적인(ancillary) 정보를 제공하여, 가장 similarity가 높은 테이블을 증강(새로 추가)할 수 있도록 한다.
- https://dl.acm.org/doi/10.1145/3183713.3196888
- Web tables 사이에 latent link structure (잠재적인 링크 구조)가 있을 수 있다는 연구가 있음.
- Table similarity 관련 최근 연구→ embedding approach를 이용한 semantic similarity가 syntactic measures(구문 측정)보다 퍼포먼스를 향상시킬 수 있다.
- Zhang, S., Balog, K.: Ad hoc table retrieval using semantic similarity. In: Proceedings of the 2018 World Wide Web Conference on World Wide Web, WWW 2018, Lyon, France, April 23–27, 2018, pp. 1553–1562 (2018). https://doi.org/10.1145/3178876. 3186067
● Table completion.
- Table extension과 마찬가지로 table similarity에 의존도 높음.
- imputation(결측값 대체), 미완성 데이터 문제와 연관됨.
- Web context에서는 주로 external data를 활용하여 해결
- 태스크:
- table 기반 태스크 - 테이블에 새 행(row)을 추가, column을 참고하여 시스템이 남은 rows 채우기
- entity-set completion 태스크 - seed entities 집합이 주어졌을 때, 리스트를 채우는 것.
- entity centric search, knowledge-base completion과 같은 시나리오와 관련있음.
3_ Current dataset search Implementations
- 현존하는 Dataset search services 의 데이터셋 처리 방법에 대한 정리를 다룬 섹션.
- 데이터셋의 centralized / decentralized architecture 를 구분
- 웹, 리포지토리에서 데이터셋 검색 전략의 공통 주제:
- 정확한 포맷으로 적절한 정보를 태깅하는 작업 + 데이터 퍼블리셔 대한 의존
- 현재 데이터셋 검색은, 데이터셋의 메타데이터만 사용하므로, 메타데이터의 디스크립션이 정확하게 유지되어야 함.
- 특히, 과학 데이터셋의 경우, 더 나은 통합된 메타데이터의 생성 지원을 이니셔티브(initiative)있음.
- 예: CEDAR by the Metadata Center2, ‘Data in Brief’ submissions supported by Elsevier.3
[Table 1] Search technology used across implementations
-Tabular search는 상업적으로 쓰이고 있지 않음.
Entity-centric | Information retrieval | Database | Tabular search | |
Open data publishing platforms (e.g., CKAN, Socrata) | O | |||
Data marketplaces | O | |||
Linked data search engines | O | O | O | |
Google DSS | O | O |
3.1_ Basic, centralized search
3.1.1_ Open government data portals
● Open data portals
- 제공하는 데이터셋에 대하여 메타데이터 검색을 허용함.
- 데이터셋과 그 메타데이터는, 해당 자료의 owner가 직접 등록한다. 그렇기에 실제로는 데이터셋을 탐색할 필요가 없고, 데이터셋과 메타데이터의 설명을 제시할 필요가 없음.
- 한계
- 데이터셋의 메타데이터 퀄리티 문제: 대부분의 경우 메타데이터가 데이터의 potential을 모두 설명하지 않음. query검색 했을 때, 데이터셋 디스크립션에 해당 키워드가 없다면 검색결과에 포함되지 않음.
- keyword-based query와 메타데이터를 매칭하고 랭킹하는 기능 부족
- 예1) CKAN
- Lucene을 사용하여 문서를 인덱싱하는 Apache Solr5를 사용하여 구축
- 도큐먼트들은 CKAN으로 표기된 퍼블리셔들이 제공하는 데이터셋의 메타데이터 들이다.
- 예2) 이 외, Socrata, OpenDataSoft 등
3.1.2_ Enterprise search
- 아키텍쳐 관점에서 보면, Proprietary data portals(독점 데이터 포털)도 비슷함.
- Google의 Goods (2016)
- Enterprise dataset search system
- 회사 내 여러 부서에서 가져온 데이터셋을 관리하기 위한 목적 (데이터 카탈로그)
- 데이터셋의 구조 또는 수집 빈도를 기반으로 데이터셋의 클러스터를 나눔.
- https://research.google/pubs/pub45390/
3.1.3_ Scientific data portals
- 여러 상용 포털에서 과학 데이터셋에 대한 엑세스 제공
- 동작방식은 다른 유형 시스템과 비슷
- 데이터 퍼블리셔가 컴파일한 centralized 데이터셋 풀의 메타데이터 레코드에 대하여, keyword-based 또는 faceted search를 제공
- 서비스 예: Elsevier [57], Figshare [171] 및 Dataverse [5]
- (offering keyword- or faceted search over metadata records of a centralized pool of datasets that is compiled with the help of data publishers. )
3.1.4_ Data marketplaces
- 특정 조직이 그의 데이터의 가치를 실현하기 위한 방법
- 검색 태스크: 사용자의 query와 데이터셋 디스크립션을 매칭한다.
- 데이터셋 디스크립션은 accessibility 또는 가격과 관련된 맞춤형(bespoke) 메타데이터 attributes를 포함한다.
- 결과를 계산하지 않고, 데이터값의 추정치를 사용자에게 전달할 수 있는 query handling 접근방식을 찾는 것이 챌린지.
3.2_ Basic, decentralized search
3.2.1_ Search over linked data
- linked data는 web sale에서의 데이터셋 검색을 용이하게 함.
- 데이터셋 사이의 링크를 따라가며 RDF data를 쿼리된 데이터셋에 지속적으로 추가함으로써, 쿼리를 실행하는 중에 새로운 데이터셋이 발견됨.
- 의미론적으로 이질적이고(heterogeneous), 분산된 환경에서 linked data search에 관련된 연구들이 있음.
3.2.2_ Google Dataset Search
- Google의 Dataset Search (2018)
- google은 웹 상의 데이터셋을 검색하는데 맞춤화된 vertical web search engine을 소개.
- Google Web Crawl을 기반으로, schema.org 데이터셋 클래스 및 DCAT을 사용해 설명된 모든 데이터셋에 대해 웹을 크롤링하고, 관련 메타데이터를 수집함.
- 메타데이터를 다른 리소스에 연결하고, 복제본을 식별하고, 각 데이터셋에 대한 풍부한 메타데이터 인덱스를 생성.
- 메타데이터는 google knowledge graph에 포팅(reconcile)되고, 검색 기능은 이 메타데이터 위에 구축된다.
- 인덱싱된 데이터셋은 keywords와 CQL 표현식을 통해 쿼리된다.
3.2.3_ Domain-specific search
- 몇 검색 서비스는 특정 도메인에 초점을 둠.
- 데이터셋을 설명하기 위한 맞춤형 메타데이터 스키마를 제시하고, 크롤러를 구현하여 자동으로 검색(탐색)한다.
- 예를 들어: DataMed (biomedical search engine) - DATS라는 태그모음을 사용하여 크롤러가 자동으로 과학데이터셋을 인덱싱할 수 있게 함.
- Open Contracting Partnership은 Open Contracting Data Standard 릴리즈.
- 크롤러가 계약 관련 데이터셋에 엑세스하고 분류(catalogue)할 수 있도록, 계약에 필요한 정보를 식별.
3.3_ Constructive search
Data가 수익을 창출할 수 있는 상품(commodity)이 될 수 있다.
- 방법1: 분산된 방식으로 존재하는 데이터를 수집하여 가치를 제공
- Thomson Reuters: 수십 년 동안 판매용 데이터셋을 위한 데이터셋 수집.
- OpenCorporates: 출처가 있는 공공데이터를 활용하여 법인(legal entities) 정보 수집 및 공개.
- Researchably: 과학관련 출판물(publications)에서 정보를 수집하여 biotech회사에 판매할 데이터셋 구축.
- 방법2: 데이터 마켓플레이스에서 데이터셋 카탈로그 판매
- 데이터셋 전체 또는 필요에 따라 하위 집합에 엑세스하여 새로운 데이터셋 구성 가능
4_ Survey of dataset search research
아래와 같은 순서로 정의
- Query Language (writing) > Query Handling > Data Handling > Results Presentation
4.1_ Querying
Creating queries
- dataset search에서는 dataset과 task의 관계가 중요하다.
- Data-centric task (사용자의 정보에 대한 니즈에 따라 두 타입이 있음.)
- (1) Process-oriented tasks: 데이터를 변형해서 사용하는 작업. (예: 머신러닝)
- 높은 우선순위: timeliness(적시성), licenses, updates, quality, methods of data collection and provenance(출처)
- (2) Goal-oriented tasks: 질문에 대한 답을 데이터에서 찾기 위한 작업.
- 높은 우선순위: 데이터의 본질적인 품질(intrinsic qualities)가 중요. 예: coverage and granularity(세분화)
Query types
데이터셋 검색을 지원하는 공식적인 query language는 없으며, 데이터셋의 metadata에 대해 keyword 또는 CQL을 사용해서 검색함.
플랫폼에 특화된 faceted search를 통해 기본적인 필터링을 할 수 있음.
4.1_ Query handling
TBD
나의 의견
논문을 선택한 이유
(1) 올해 초, '파일 검색' 이라는 주제에 관심이 있었다. 그래서 'Information Retrieval' 관련 survey 논문을 읽어보려고 하던 차에 이 논문을 발견했고, '데이터셋' 또한 파일이기 때문에 파일 검색의 하위 분야가 될 수도 있겠다고 생각했다. (넓은 의미에서 '파일'을 local 뿐만 아니라 웹 상에 올려둔 파일까지 포함한다면.)
데이터셋을 검색하는 목적이나 파일을 검색하는 목적이 '정보를 얻고자 함' 이라는 공통 목적을 포함하기 때문에 이 논문을 읽어보았다.
읽고 난 후 의견
- '검색' 이라는 분야는 UX에서도 중요한 부분인데, NLP나 Database, 결과 화면 최적화 등 기술적으로도 매우 어렵고 중요한 분야라는 생각이 들었다.
- Survey 논문이라 각 세부적인 내용을 담지는 않지만, 검색 전반에 대한 큰 개념을 알 수 있어 좋았다.